Centralized Inventory Control in a Partially Observable State#
Business objective#
The general objective of supply chain management is to provide users with high value services while dealing with the problem of inventory cost. To solve the problem, we can collect historical data and other relevant information from the customer side. At each decision step (e.g. $T_1$), the system will decide whether to replenish and also how many (optimal order) based on the observation of the current inventory status. An implicit intermediate step in this process is that the current inventory will be firstly consumed to fulfill the customer recent demand, and then replenish by the newly supply goods.
There are several factors that affect the quality of inventory management:
making an accurate forecast of future demand is one of key problems;
how accurate of the observation can reflect the actual inventory states;
whether the data collected is from a single domain or a mix of multiple domains;
a centrailized strategy is useful if exits a strong correlation among multiple commodities sales;
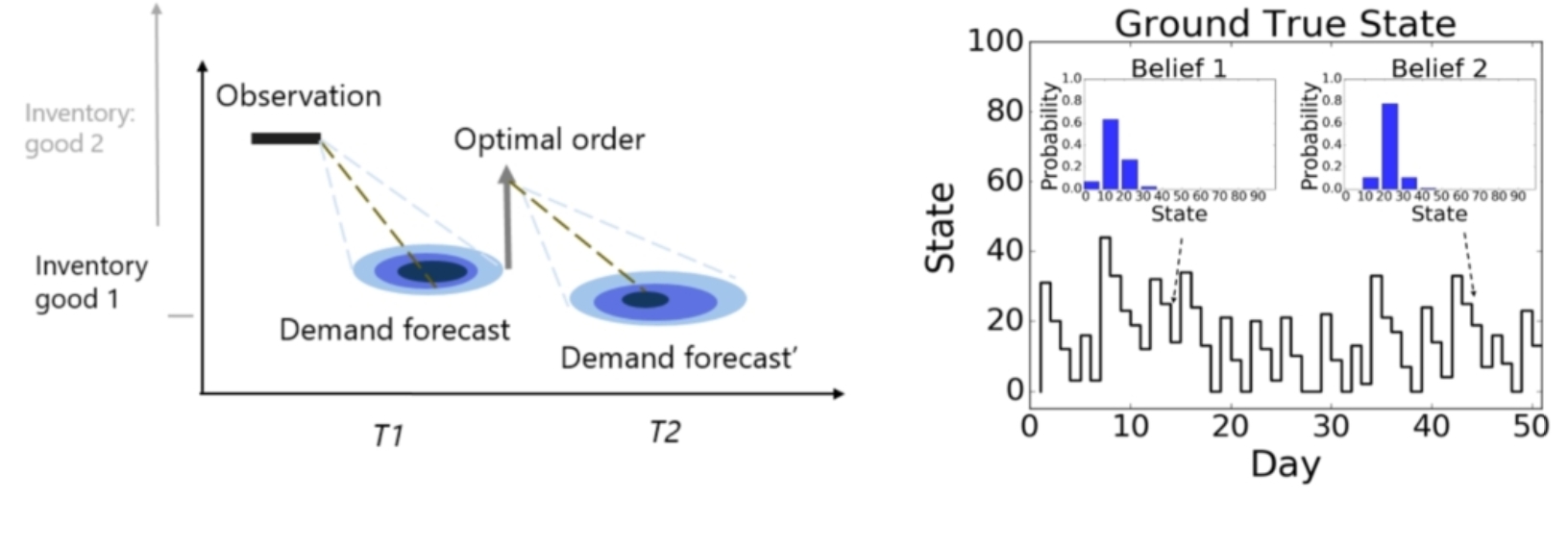
Dealing with uncertainties#
Industrial investigations indicate that errors in inventory recordings are common and often unavoidable. Such errors result in dramatic wastes and cost to the industry. Inventory control in the presence of such errors is essentially a partially-observed decision-making problems. Although robust framework, such as the Partially Observable Markov Decision Processes (POMDPs) have been applied to inventory control, most work apply POMDPs to single commodity problems or assume independence between commodities, due to difficulties in solving problems with large discrete action space. This work [1] applies our method, QBASE, to problems with multiple commodities whose demand levels may be correlated. Numerical experiments on partially observed multi-commodity inventory control problems indicate that our proposed solution can find less conservative inventory control strategies than benchmark methods do. Specifically, QBASE can generate a better policy, whereby a small amount of sales are sacrificed to keep the inventory level of commodities with expensive storage cost and low value, to be as low as possible, which then lead to a higher profit
Left: Average total discounted reward, which reflects the average total profit, as time increases.
Right: The inventory levels maintained for each type of commodity. Higher inventory level indicates a more conservative strategy.
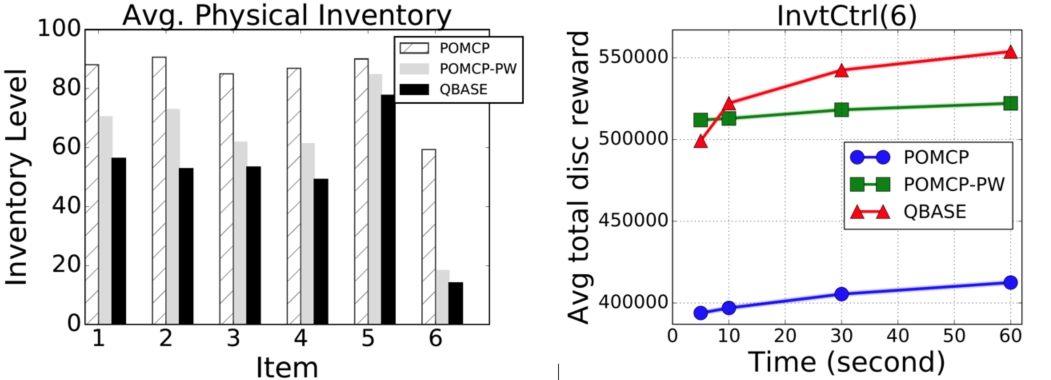
A simulation run#
Here’s an animation of how the inventory works at each time point.
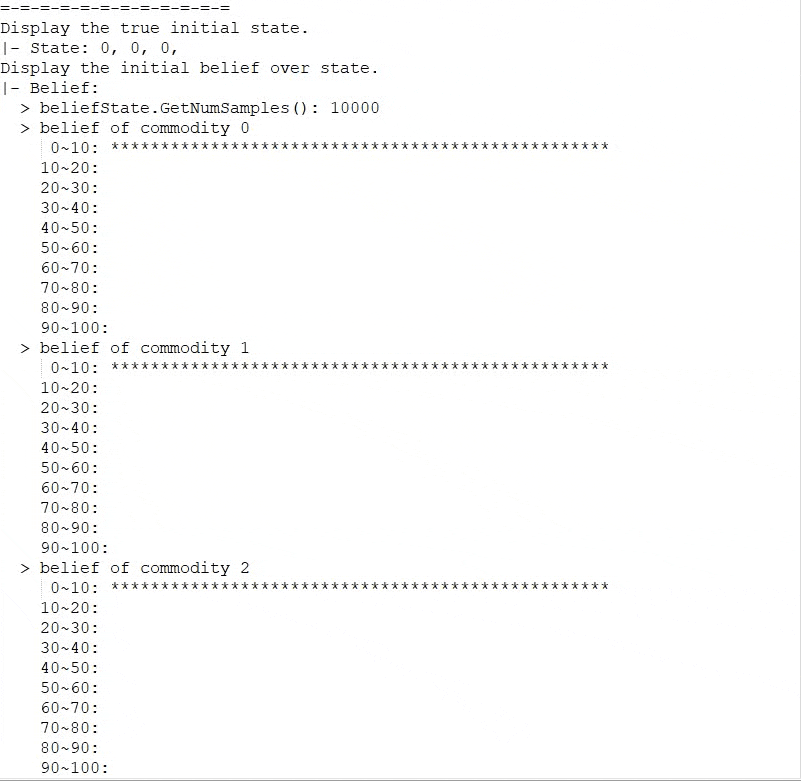
Reference
Erli Wang and Hanna Kurniawati and Dirk Kroese. An On-line Planner for POMDPs with Large Discrete Action Space: A Quantile-Based Approach. Proc. Int. Conference on Automated Planning and Scheduling (ICAPS). 2018. (pdf,)
Erli Wang and Hanna Kurniawati and Dirk Kroese. Inventory Control with Partially Observable States. Proc. Int. International Congress on Modelling and Simulation (MODSIM). 2019. (pdf)